Wenjing Liao Awarded DOE Early Career Award for Model Simplification, Deep Learning
Oct 03, 2023 —
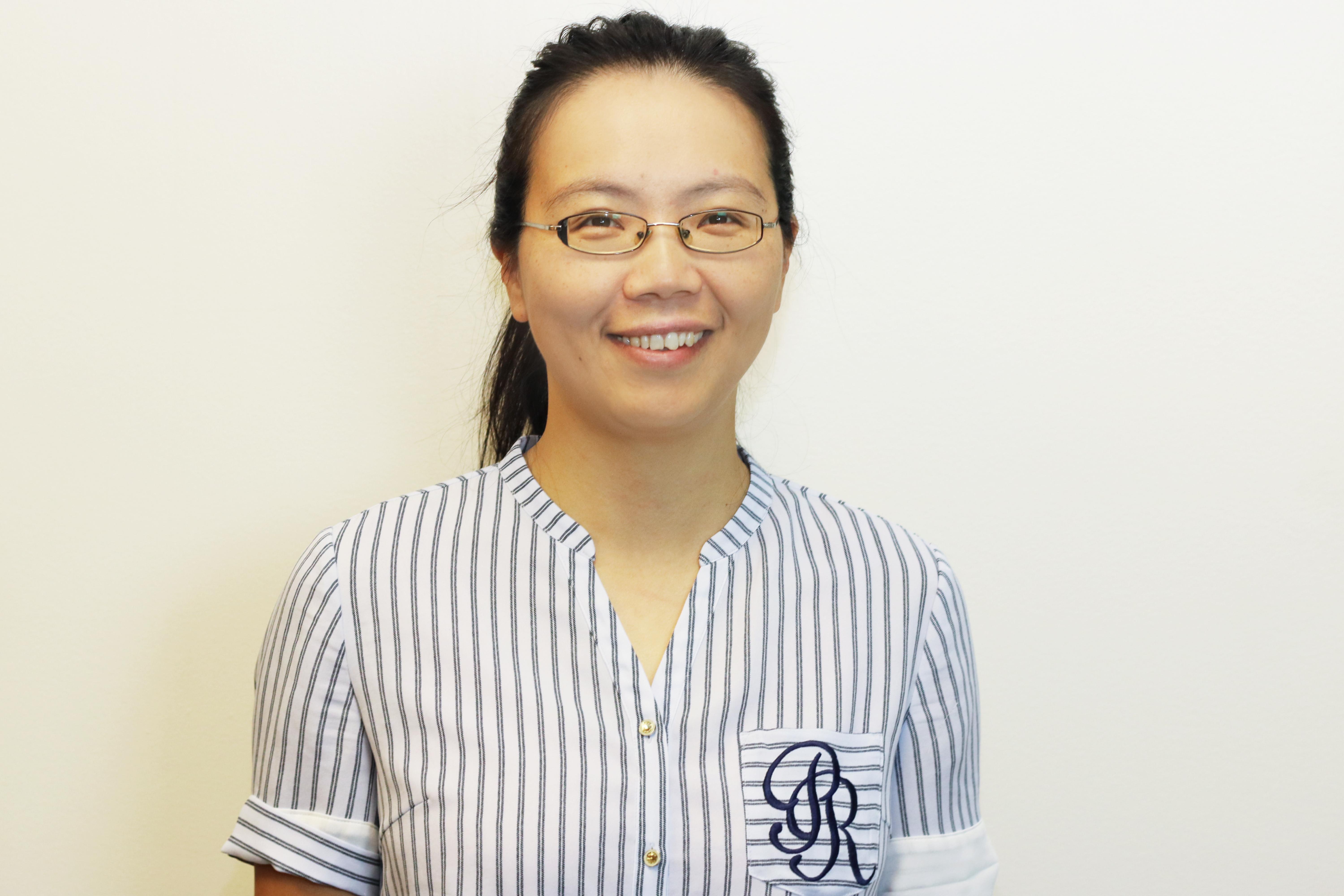
Wenjing Liao, an associate professor in the School of Mathematics, has been awarded a Department of Energy (DOE) Early Career Award for her research into how deep learning might be leveraging to make mathematical advances in achieving more efficient modeling techniques.
Liao was selected as one of the 93 early career scientists from across the country who are receiving a combined $135 million in DOE funding. The awards aim to support the next generation of STEM leaders, and identify early-career scientists whose research will have global impacts.
Earlier this year, Liao was also selected for an National Science Foundation (NSF) Faculty Early Career Development Program (CAREER) Award, one of the most prestigious grants that a scientist can receive early in their profession.
“Supporting America’s scientists and researchers early in their careers will ensure the U.S. remains at the forefront of scientific discovery and develops the solutions to our most pressing challenges,” said U.S. Secretary of Energy Jennifer M. Granholm, adding that the funding “will allow the recipients the freedom to find the answers to some of the most complex questions as they establish themselves as experts in their fields.”
Model simplification; complex problems
Real-world applications of computer modeling often call for large, complex data simulations, which can be time-consuming and expensive, limiting their applications. Liao’s project “Model Reduction by Deep Learning: Interpretability and Mathematical Advances” focuses on a technique called model reduction, which allows researchers to reduce the size of problems computer models must solve to smaller ones that computers can efficiently solve.
Liao notes that while traditional model-reduction methods have been successful, the technique is mostly limited to low dimensional linear models, or those with fewer important features that the model can include. However, many problems found in nature are the opposite. Liao hopes that by identifying the underlying nonlinear structures in natural problems, she can broaden the application of model-reduction techniques.
To do so, her research will focus on three key questions. First, she will investigate how to leverage deep neural networks to extract low-dimensional nonlinear structures in data sets. Next, Liao will investigate how to use the nonlinear structures in model reduction. Finally, in order to better harness deep learning, Liao aims to develop new deep learning-based model reduction methods.
“This project has the potential to drive significant advances in scientific machine learning,” Liao says in her abstract. “The proposed model-reduction methods can be used to analyze large datasets and simulate complex phenomena in physics, biology, and engineering.”
Written by Selena Langner