Research Finds Models Used to Detect Malicious Users on Popular Social Sites are Vulnerable to Attack
Nov 22, 2021 — Atlanta, GA
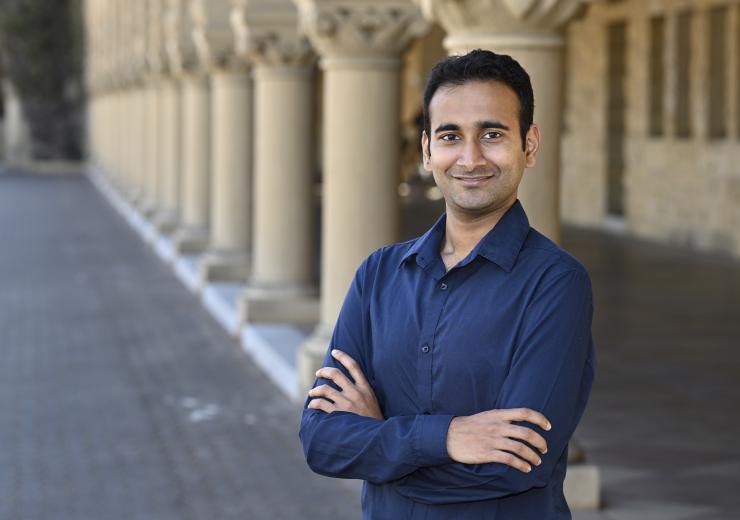
Whether they are fake accounts, sock puppet accounts, spammers, fake news spreaders, vandal editors, or fraudsters, malicious users pose serious challenges to the security of nearly every virtual platform.
Now, research led by Georgia Tech has identified a new threat to deep learning models used to detect malicious users on Facebook and other popular e-commerce, social media, and web platforms.
“Social media and web platforms put a lot of energy, effort, and resources into creating state-of-the-art methods to keep their platforms safe," said Srijan Kumar, School of Computational Science and Engineering assistant professor and co-investigator.
"To do this, they create machine learning and artificial intelligence models that essentially try to identify and separate malicious and at-risk users from the benign users.”
The new attack model generates text posts that mimic a personalized writing style and that are inflected by contextual knowledge of a given target site. The generated posts also are informed by the historical use of a target site and recent topics of interest.
Known as PETGEN, the work represents the first time researchers have successfully conducted adversarial attacks on deep user sequence classification models.
“There is always this game between the models trying to catch the malicious users and the accounts trying to not get caught. If we can act as attackers, we can identify the model vulnerabilities and the potential ways these malicious accounts evade detection systems,” Kumar said.
The research was conducted on two real-world datasets from Yelp and Wikipedia. Although the PETGEN research reveals that malicious users can evade these types of detection models, Kumar says he and his team are working now to develop a defense against this type of attack.
“The findings will help to pave the path toward the next generation of adversary-aware sequence classification models and better safety for virtual interactions on these platforms,” Kumar said.
The findings of this work were first presented at the 27th ACM SIGKDD Conference on Knowledge Discovery and Data Mining by Kumar, School of Computer Science Professor Mustaque Ahamad, and computer science Ph.D. student Bing He.
The work presented in PETGEN is also an output of a Facebook research award that Kumar received jointly with CSE Associate Professor Polo Chau.
Albert Snedeker, Communications Manager II
albert.snedeker@cc.gatech.edu