Group Brings Seismic Imaging Studies to Conversations on Climate Change and Beyond
Aug 08, 2022 — Atlanta, GA
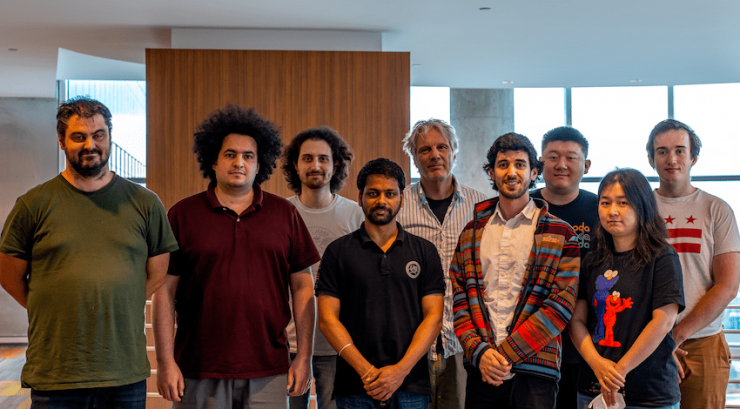
A summer dominated by high gas prices and record-setting temperatures around the globe has intensified discussions on climate change. At the same time, a Georgia Tech research group has spent this summer preparing studies that could help reduce greenhouse gas levels through machine learning and high-performance computing methods.
The School of Computational Science and Engineering’s (CSE) Seismic Laboratory for Imaging and Modeling (SLIM) is a research group that specializes in providing industry partners with computational models that advance seismic imaging. Led by Professor Felix J. Herrmann, one area SLIM devotes their imaging research toward is Geological Carbon Storage (GCS), an emerging solution to help combat climate change.
“SLIM has been widely recognized as a world leader in the next generation of seismic acquisition, data processing, imaging, and monitoring for the oil and gas industry,” said Herrmann, who is based in the School of Earth and Atmospheric Sciences and holds a joint appointment with the School of CSE. “SLIM has recently made developments in the fields of compressive sensing and machine learning to drive innovations in wave-based inversion with applications in seismic monitoring.”
GCS is a process of removing carbon dioxide from the atmosphere and storing it in deep, underground reservoirs. SLIM’s research in seismic imaging assists engineers to monitor carbon dioxide dynamics stored in the Earth’s subsurface. This includes detecting potential leaks in underground reservoirs, which minimizes risks in GCS projects.
While GCS is one of the few truly scalable solutions to help combat climate change, challenges remain to increase the number of injections sites while reducing risks and cost. These challenges make SLIM’s studies even more important for areas that stand to benefit from time-lapse seismic imaging, like GCS.
One obstacle inhibiting GCS is difficulty in conducting large-scale seismic imaging that is accurate, timely, and cost-effective. SLIM provides a novel approach that maps seismic images associated with one background model to another through velocity continuation. SLIM’s velocity continuation method is cheaper and faster than existing algorithms because it avoids creating new images from scratch. This potentially opens the way for large-scale, uncertainty-aware monitoring.
Another challenge in monitoring GCS is predicting how stored carbon dioxide will behave underground due to reservoir fluid properties like temperature and pressure. To address this problem, SLIM developed a model that uses a neural operator in place of a fluid-flow simulator. According to this study, the neural operator can forecast behavior of stored carbon dioxide at a fraction of computational cost of conventional numerical simulations.
A similarity between these studies are their use of Fourier neural operators. A neural operator is a deep learning method that solves partial differential equations, the essential mathematical formulations in understanding engineering phenomena. Fourier neural operators are a class of neural operator that once trained, can produce nearly identical results at several orders of magnitude faster compared to traditional numerical solvers.
“By gradually shifting gear to carbon storage monitoring with seismic techniques, SLIM aims to be part of the solution to climate change,” said CSE Ph.D. student Ziyi (Francis) Yin. “With the recent innovations in the group, we want to lower risk of carbon storage projects.”
Studying geophysical phenomenon without simulations is a SLIM specialty. Another SLIM paper introduces a simulation-free seismic survey design. SLIM’s method uses an annealing algorithm that provides accurate wavefield reconstruction with minimal seismic survey data. This design improves seismic data reconstruction without expensive and time-consuming wavefield simulations
SLIM tested their research at small scale using synthetic case studies. The studies introduce solutions to seismic imaging and carbon sequestration that can be replicated at large scale and are available for industry use due to their simplicity and cost-effectiveness. Even more, their methods have other potential applications such as further seismic exploration, medical imaging, and high-performance computing.
SLIM will present these studies Aug. 28 – Sept. 1 at the upcoming International Meeting for Applied Geoscience and Energy in Houston. IMAGE’22 provides a venue for geoscientists, industry professionals, and thought leaders to share best practices and develop strategies for the future, to include carbon sequestration.
“We have several presentations scheduled covering topics such as graph-theory-based seismic survey design to deep-learning-based methods for seismic imaging and uncertainty quantification,” said CSE alumnus Ali Siahkoohi. Siahkoohi completed his Ph.D. this summer and is now a postdoctoral scholar at Rice University.
Much of SLIM’s research funding comes from the Center for Machine Learning for Seismic Industry Partners Program (ML4SEISMIC). ML4SEISMIC is an initiative co-led by SLIM and Georgia Tech’s Center for Energy and Geo Processing that fosters research partnerships with innovators in the energy sector.
“SLIM has been involved with Society of Exploration Geophysics conferences for many years. Every year, we bring our latest developments to the IMAGE conference,” Yin said. “With the talent in our group, we can tackle large-scale geophysical problems with our cutting-edge computational methods.”
Bryant Wine, Communications Officer
bryant.wine@cc.gatech.edu