Artificially Intelligent Neural Interfaces
Jul 15, 2019 — Atlanta, GA
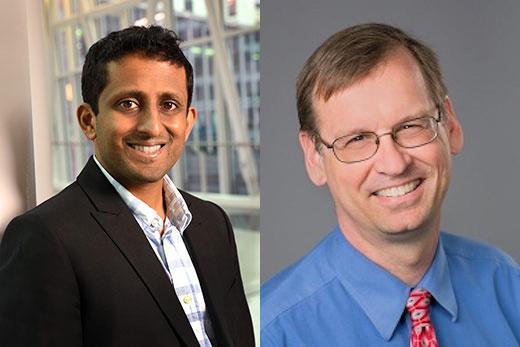
Chethan Pandarinath and Lee Miller were awarded a $1 million grant from DARPA for their research on artificial intelligence and neural interfaces.
Paralyzed people moving their limbs or operating prosthetic devices by having machines decipher the electrical impulses in their nervous systems: it’s an appealing vision, and one that is getting closer. Right now, when a computer “reads” someone’s brain, the interface between brain and machine does not stay the same, so the computer needs to be recalibrated once or multiple times a day. It’s like learning to use a tool, and having the weight and shape of the tool change.
To address this challenge, biomedical engineers at Emory and Georgia Tech, working with colleagues at Northwestern University, were awarded a $1 million grant from DARPA (Defense Advanced Research Projects Agency). The two-phase grant begins with $400,000 for six months, and can advance to a total of $1 million over 18 months.
Chethan Pandarinath and Lee Miller are combining artificial intelligence-based approaches that their laboratories have developed that enable the decoding of complex signals from the nervous system controlling movement. The scientists plan to develop algorithms that periodically and automatically recalibrate so that nervous system “intent” can be decoded smoothly and without interruption.
Pandarinath and Miller’s project is being funded under DARPA’s $2 billion AI Next campaign, which includes a “high-risk, high-payoff” Artificial Intelligence Exploration program. DARPA officials see the campaign as part of a “third wave” of artificial intelligence research. The first wave focused on rule-based systems capable of narrowly defined tasks. The second wave, beginning in the 1990s, created statistical pattern recognizers from large amounts of data, which are capable of impressive feats of language processing, navigation and problem solving. However, they do not adapt to changing conditions, offer limited performance guarantees, and are unable to provide users with explanations of their results. The third wave, in contrast, will focus on contextual adaptation and enabling machines to function reliably despite massive volumes of changing or even incomplete information.
Pandarinath, a researcher in the Petit Institute for Bioengineering and Bioscience at Georgia Tech, is an assistant professor in the Wallace H. Coulter Department of Biomedical Engineering at Georgia Tech and Emory (and part of Emory's Department of Neurosurgery and Neuromodulation Technology Innovation Center). Miller is a professor of physiology, physical medicine & rehabilitation, and biomedical engineering at Northwestern University. Pandarinath and Miller have an already established collaboration, and are part of a National Science Foundation-funded project on building new approaches to handle data from the nervous system at unprecedented scale.
Pandarinath and colleagues previously developed an approach that uses artificial neural networks to decipher the complex patterns of activity in biological networks that make our everyday movements possible. Prior approaches focused on the activity of individual neurons in the brain, and attempted to relate their activity to movement variables like arm speed, movement distance or angle.
Instead, Pandarinath says, patterns that are spread out across the entire network are far more important, and uncovering these distributed patterns is the key to breakthroughs in neural interface technologies. The distributed patterns, or “manifolds,” are highly stable, lasting for months or years. Thus manifolds could provide a stable platform when engineers are seeking to build prosthetics or neural interface-controlled devices that can restore movement abilities for paralyzed people across months and years without needing any sort of manual recalibration.
Pandarinath’s manifold decoding approach will be combined with a separate neural network approach called ANMA (Adversarial Neural Manifold Alignment), developed by Miller’s team, which can adjust the manifolds to any changes in the incoming data. Together, Pandarinath and Miller call their combined technology NoMAD, for Nonlinear Manifold Alignment Decoding.
Their experiments will be based on data that has already been collected in non-human primates, in which monkeys control an on-screen cursor via wrist movements, or perform various natural behaviors. To test the resilience of their technology, the scientists plan to incorporate instabilities into their experiments, which would simulate the effects of shifting an electrode or changes in physiological conditions.
The scientists say that NoMAD will be applicable to a wide variety of neural interfaces, since manifolds integrate neural patterns in the motor, sensory and cognitive realms. Thus, beyond prosthetic devices and movement control, NoMAD could eventually refine and improve electrical stimulation therapies for Parkinson’s, epilepsy, speech, depression, or psychiatric disorders.
Quinn Eastman
404-727-7829
qeastma@emory.edu