AI and Automation Converge in Expansion of Georgia Tech’s Advanced Manufacturing Pilot Facility
Jul 23, 2024 —
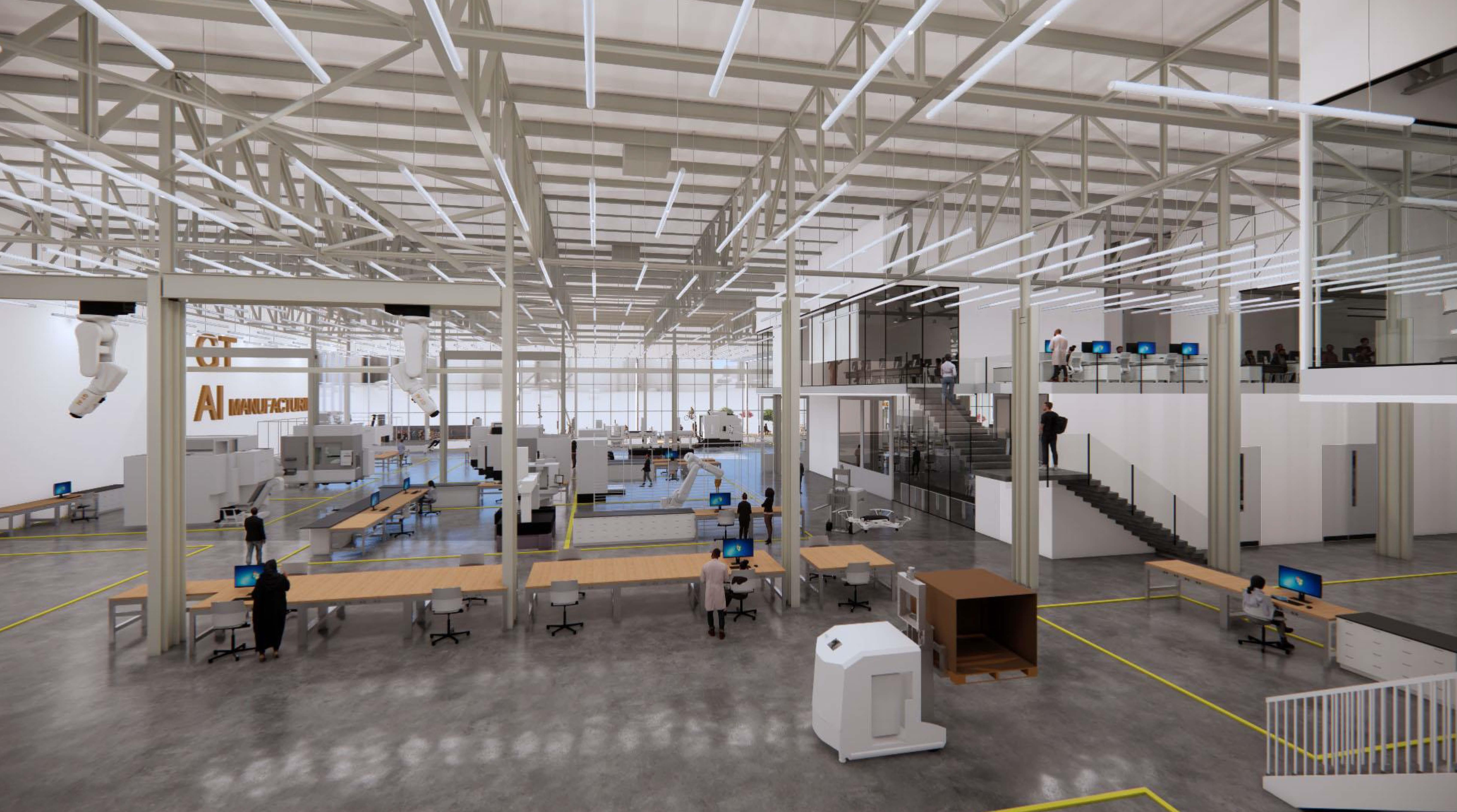
An early rendering of the main expanded research area at the Advanced Manufacturing Pilot Facility (Credit: Lord Aeck Sargent).
When it comes to manufacturing innovation, the “valley of death” — the gap between the lab and the industry floor where even the best discoveries often get lost — looms large.
“An individual faculty’s lab focuses on showing the innovation or the new science that they discovered,” said Aaron Stebner, professor and Eugene C. Gwaltney Jr. Chair in Manufacturing in the George W. Woodruff School of Mechanical Engineering. “At that point, the business case hasn't been made for the technology yet — there's no testing on an industrial system to know if it breaks or if it scales up. A lot of innovation and scientific discovery dies there.”
The Georgia Tech Manufacturing Institute (GTMI) launched the Advanced Manufacturing Pilot Facility (AMPF) in 2017 to help bridge that gap.
Now, GTMI is breaking ground on an extensive expansion to bring new capabilities in automation, artificial intelligence, and data management to the facility.
“This will be the first facility of this size that's being intentionally designed to enable AI to perform research and development in materials and manufacturing at the same time,” said Stebner, “setting up GTMI as not just a leader in Georgia, but a leader in automation and AI in manufacturing across the country.”
AMPF: A Catalyst for Collaboration
Located just north of Georgia Tech’s main campus, APMF is a 20,000-square-foot facility serving as a teaching laboratory, technology test bed, and workforce development space for manufacturing innovations.
“The pilot facility,” says Stebner, “is meant to be a place where stakeholders in academic research, government, industry, and workforce development can come together and develop both the workforce that is needed for future technologies, as well as mature, de-risk, and develop business cases for new technologies — proving them out to the point where it makes sense for industry to pick them up.”
In addition to serving as the flagship facility for GTMI research and the state’s Georgia AIM (Artificial Intelligence in Manufacturing) project, the AMPF is a user facility accessible to Georgia Tech’s industry partners as well as the Institute’s faculty, staff, and students.
“We have all kinds of great capabilities and technologies, plus staff that can train students, postdocs, and faculty on how to use them,” said Stebner, who also serves as co-director of the GTMI-affiliated Georgia AIM project. “It creates a unique asset for Georgia Tech faculty, staff, and students.”
Bringing AI and Automation to the Forefront
The renovation of APMF is a key component of the $65 million grant, awarded to Georgia Tech by the U.S. Department of Commerce’s Economic Development Administration in 2022, which gave rise to the Georgia AIM project. With over $23 million in support from Georgia AIM, the improved facility will feature new workforce training programs, personnel, and equipment.
Set to complete in Spring 2026, the Institute’s investment of $16 million supports construction that will roughly triple the size of the facility — and work to address a major roadblock for incorporating AI and automation into manufacturing practices: data.
“There’s a lot of work going on across the world in using machine learning in engineering problems, including manufacturing, but it's limited in scale-up and commercial adoption,” explained Stebner.
Machine learning algorithms have the potential to make manufacturing more efficient, but they need a lot of reliable, repeatable data about the processes and materials involved to be effective. Collecting that data manually is monotonous, costly, and time-consuming.
“The idea is to automate those functions that we need to enable AI and machine learning” in manufacturing, says Stebner. “Let it be a facility where you can imagine new things and push new boundaries and not just be stuck in demonstrating concepts over and over again.”
To make that possible, the expanded facility will couple AI and data management with robotic automation.
“We're going to be able to demonstrate automation from the very beginning of our process all the way through the entire ecosystem of manufacturing,” said Steven Sheffield, GTMI’s senior assistant director of research operations.
“This expansion — no one else has done anything like it,” added Steven Ferguson, principal research scientist with GTMI and managing director of Georgia AIM. “We will have the leading facility for demonstrating what a hyperconnected and AI-driven manufacturing enterprise looks like. We’re setting the stage for Georgia Tech to continue to lead in the manufacturing space for the next decade and beyond.”
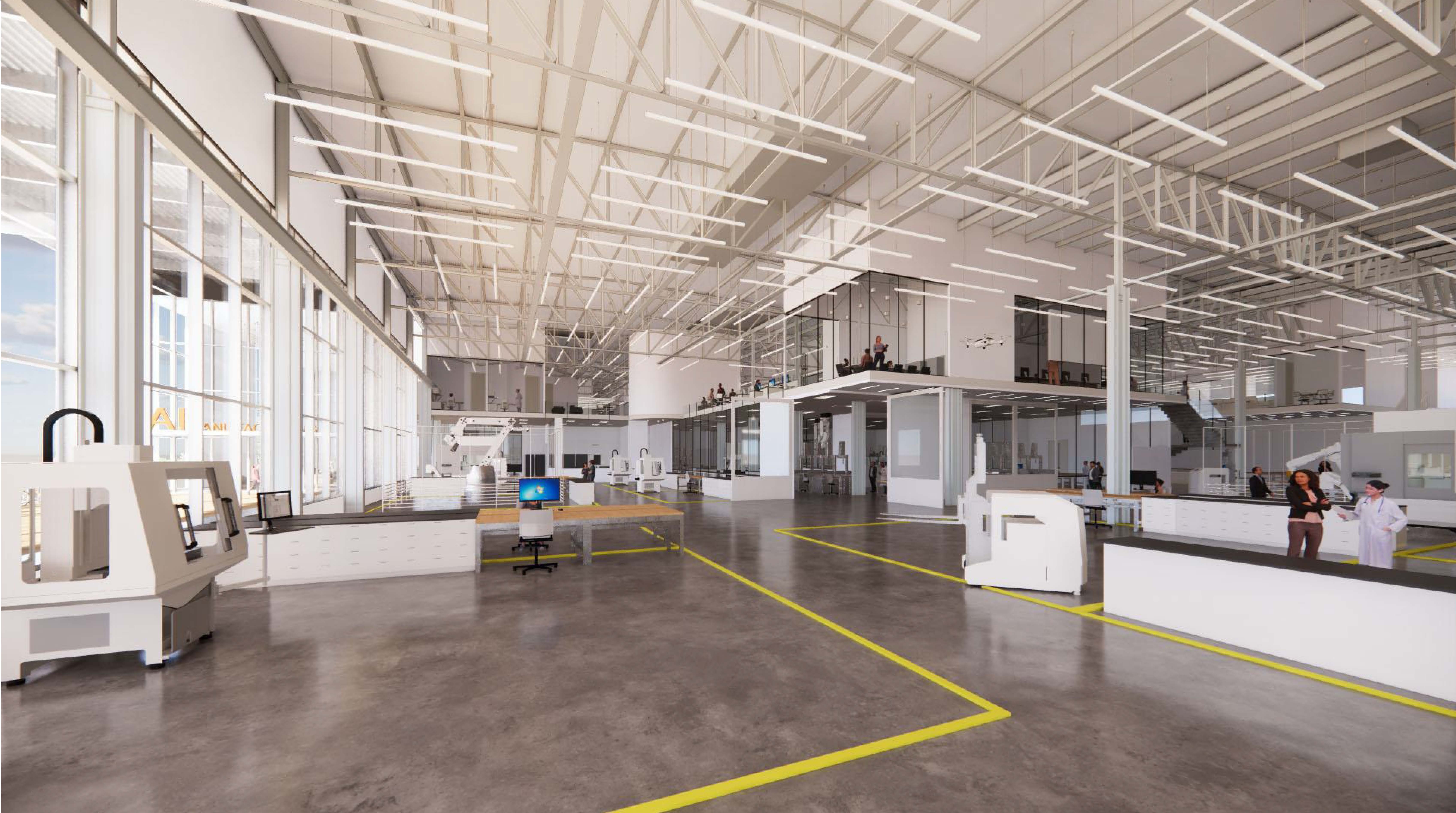
An early rendering of the main expanded research area at the Advanced Manufacturing Pilot Facility (Credit: Lord Aeck Sargent).
Audra Davidson
Research Communications Program Manager
Georgia Tech Manufacturing Institute
Mechanical Engineering Researchers Use Salt for Thermal Energy Storage
Jul 23, 2024 — Atlanta, GA
Erik Barbosa and Madeline Morrell examine salt beads. Photo by: Allison Carter
From keeping warm in the winter to doing laundry, heat is crucial to daily life. But as the world grapples with climate change, buildings’ increasing energy consumption is a critical problem. Currently, heat is produced by burning fossil fuels like coal, oil, and gas, but that will need to change as the world shifts to clean energy.
Georgia Tech researchers in the George W. Woodruff School of Mechanical Engineering (ME) are developing more efficient heating systems that don’t rely on fossil fuels. They demonstrated that combining two commonly found salts could help store clean energy as heat; this can be used for heating buildings or integrated with a heat pump for cooling buildings.
The researchers presented their research in “Thermochemical Energy Storage Using Salt Mixtures With Improved Hydration Kinetics and Cycling Stability,” in the Journal of Energy Storage.
Reaction Redux
The fundamental mechanics of heat storage are simple and can be achieved through many methods. A basic reversible chemical reaction is the foundation for their approach: A forward reaction absorbs heat and then stores it, while a reverse reaction releases the heat, enabling a building to use it.
ME Assistant Professor Akanksha Menon has been interested in thermal energy storage since she began working on her Ph.D. When she arrived at Georgia Tech and started the Water-Energy Research Lab (WERL), she became involved in not only developing storage technology and materials but also figuring out how to integrate them within a building. She thought understanding the fundamental material challenges could translate into creating better storage.
“I realized there are so many things that we don't understand, at a scientific level, about how these thermo-chemical materials work between the forward and reverse reactions,” she said.
The Superior Salt
The reactions Menon works with use salt. Each salt molecule can hold a certain number of water molecules within its structure. To instigate the chemical reaction, the researchers dehydrate the salt with heat, so it expels water vapor as a gas. To reverse the reaction, they hydrate the salt with water, forcing the salt structure’s expansion to accommodate those water molecules.
It sounds like a simple process, but as this expansion/contraction process happens, the salt gets more stressed and will eventually mechanically fail, the same way lithium-ion batteries only have so many charge-discharge cycles.
“You can start with something that's a nice spherical particle, but after it goes through a few of these dehydration-hydration cycles, it just breaks apart into tiny particles and completely pulverizes or it overhydrates and agglomerates into a block,” Menon explained.
These changes aren’t necessarily catastrophic, but they do make the salt ineffective for long-term heat storage as the storage capacity decreases over time.
Menon and her student, Erik Barbosa, a Ph.D. student in ME, began combining salts that react with water in different ways. After testing six salts over two years, they found two that complemented each other well. Magnesium chloride often fails because it absorbs too much water, whereas strontium chloride is very slow to hydrate. Together, their respective limitations can mutually benefit each other and lead to improved heat storage.
“We didn't plan to mix salts; it was just one of the experiments we tried,” Menon said. “Then we saw this interactive behavior and spent a whole year trying to understand why this was happening and if it was something we could generalize to use for thermal energy storage.”
The Energy Storage of the Future
Menon is just beginning with this research, which was supported by a National Science Foundation (NSF) CAREER Award. Her next step is developing the structures capable of containing these salts for heat storage, which is the focus of an Energy Earthshots project funded by the U.S. Department of Energy’s (DOE) Office of Basic Energy Sciences.
A system-level demonstration is also planned, where one solution is filling a drum with salts in a packed bed reactor. Then hot air would flow across the salts, dehydrating them and effectively charging the drum like a battery. To release that stored energy, humid air would be blown over the salts to rehydrate the crystals. The subsequently released heat can be used in a building instead of fossil fuels. While initiating the reaction needs electricity, this could come from off-peak (excess renewable electricity) and the stored thermal energy could be deployed at peak times. This is the focus of another ongoing project in the lab that is funded by the DOE’s Building Technologies Office.
Ultimately, this technology could lead to climate-friendly energy solutions. Plus, unlike many alternatives like lithium batteries, salt is a widely available and cost-effective material, meaning its implementation could be swift. Salt-based thermal energy storage can help reduce carbon emissions, a vital strategy in the fight against climate change.
“Our research spans the range from fundamental science to applied engineering thanks to funding from the NSF and DOE,” Menon said. “This positions Georgia Tech to make a significant impact toward decarbonizing heat and enabling a renewable future.”
Erik Barbosa and Madeline Morrell (PhD students) analyze water vapor storage and release in salts. Photo by: Allison Carter
Michael Adams (postdoc) and Erik Barbosa discuss a heat and mass transfer model for a packed bed reactor of salts. Photo by: Allison Carter
The thermal energy storage team in Menon's lab. Photo by: Allison Carter
Tess Malone, Senior Research Writer/Editor
tess.malone@gatech.edu
AI4OPT and PSR Partner to Transform US Energy Industry
Jul 22, 2024 — Atlanta, GA

This partnership in the advancement of AI and mathematical optimization to address pressing energy transformations in Latin America and the U.S. has formed between the NSF Artificial Intelligence (AI) Research Institute for Advances in Optimization (AI4OPT) at Georgia Tech and PSR, Inc. - Energy Consulting and Analytics.
PSR is a global leader in analytical solutions for the energy sector, providing innovative technical consultancy services and state-of-the-art power systems planning software. Their tools are used for detailed modeling of entire countries or regions and are utilized in over 70 countries. Together with AI4OPT, they aim to leverage advancements in AI and mathematical optimization to address pressing energy transformations in Latin America and the U.S.
Latin America boasts abundant renewable energy resources, especially hydropower, leading to one of the largest shares of renewables in its energy mix. However, expanding renewable energy capacity in Latin America and the U.S. to meet decarbonization goals will require system operational advances and new technologies that can adapt to current needs.
One focus of this collaboration will be studying how to efficiently incorporate pumped storage into the resource mix as a solution for long-duration storage. These plants act as large batteries, pumping water to higher reservoirs during low demand periods and generating electricity during high demand with minimal energy loss over time. This technology supports both short-term and long-term energy storage, making it crucial for managing the variability of intermittent renewables like solar and wind.
The complex and large-scale nature of the expansion problem, exacerbated by inherent uncertainty and time-coupled decisions, traditionally requires sophisticated optimization techniques. AI innovations now provide faster solutions and better representations of non-linear dynamics, leading to more cost-effective operations and optimized energy mix selection for the energy transition.
This collaboration plans to use machine learning to enhance power system operators' ability to perform faster security checks and screenings. As renewable energy sources introduce more variability, traditional methods struggle with the increasing number of scenarios needed for grid stability. Machine learning offers a solution by expediting these analyses, supporting the integration of more renewable energy into the system.
About PSR
PSR is a global provider of analytical solutions for the energy sector, spanning from insightful and innovative technical consultancy services to state-of-the-art specialized power systems planning software applied to the detailed modelling of entire countries or regions. Having its products applied in over 70 countries, PSR contributes to the research and development of optimization and data analytics’ tools for guaranteeing a reliable and least-cost operation of power systems, helping the countries achieve their decarbonization targets.
About AI4OPT
The Artificial Intelligence (AI) Research Institute for Advances in Optimization, or AI4OPT, aims to deliver a paradigm shift in automated decision-making at massive scales by fusing AI and Mathematical Optimization (MO) to achieve breakthroughs that neither field can achieve independently. The Institute is driven by societal challenges in energy, logistics and supply chains, resilience and sustainability, and circuit design and control. To address the widening gap in job opportunities, the Institute delivers an innovative longitudinal education and workforce development program.
Breon Martin
AI Research Communications Manager
Georgia Tech
AI Hub Planned for CAU Under $2.79M NSF Grant with AI4OPT at Georgia Tech
Jul 22, 2024 —
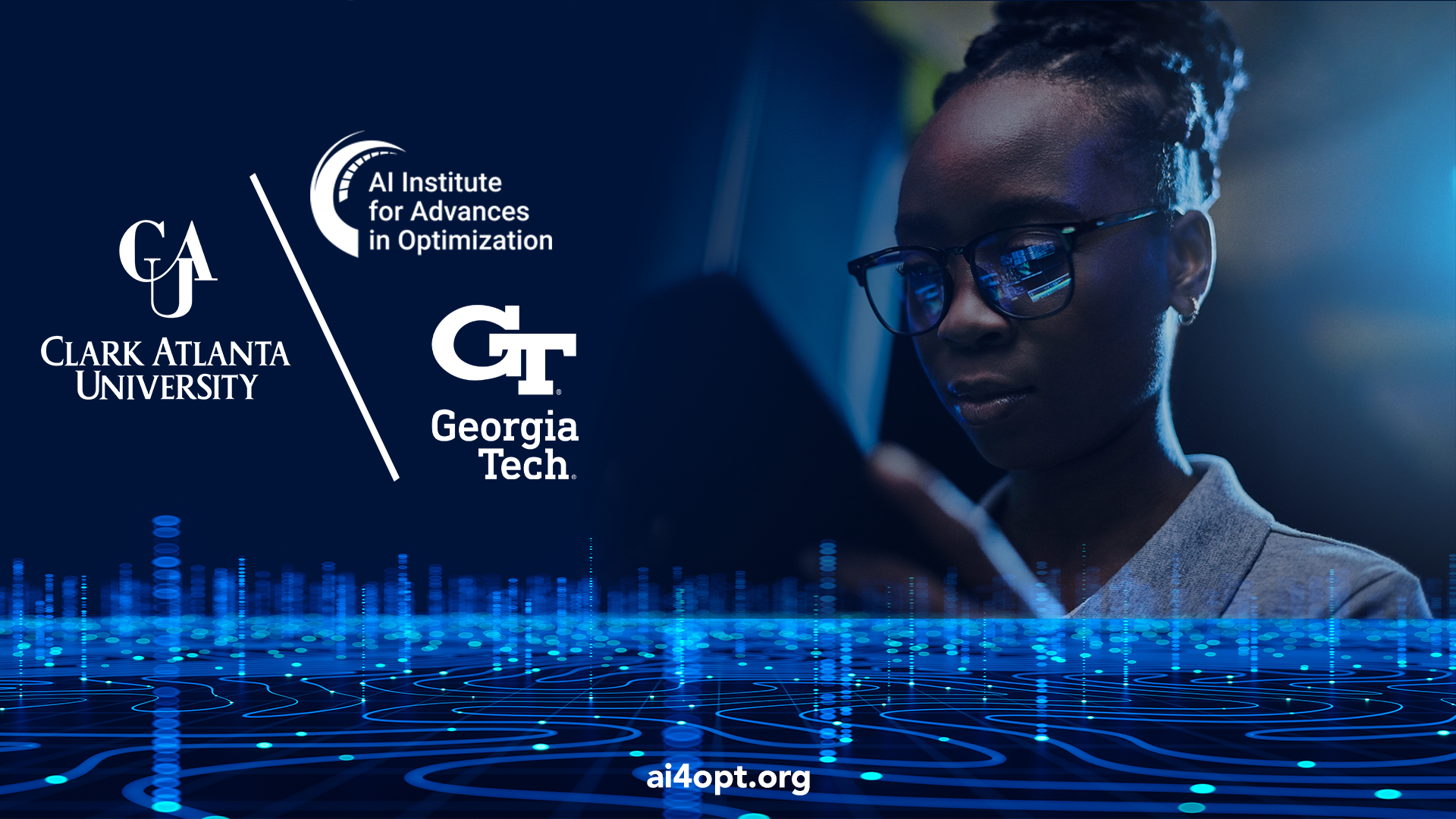
AI Hub Planned for Clark Atlanta University with $2.79M NSF Grant.
Clark Atlanta University (CAU), in collaboration with Georgia Tech’s NSF Artificial Intelligence (AI) Research Institute for Advances in Optimization (AI4OPT), has been awarded a four-year $2.79 million grant (Award ID 2402493) by the National Science Foundation (NSF) to create an AI Hub. This collaborative effort aims to advance AI education and research at minority-serving institutions, particularly historically Black colleges and universities (HBCUs).
This initiative, part of the NSF ExpandAI program, aims to boost minority-serving institution participation in AI research, education, and workforce development through capacity-building projects and partnerships within the NSF-led National AI Research Institutes ecosystem.
Building an AI community is no easy feat, but the CAU-GT/AI4OPT collaboration is prepared to meet it. The project, known as AIHUB@CAU, will be led by principal investigator Charles B. Pierre, associate professor in CAU’s Department of Mathematical Sciences.
"The mission of the grant aligns with the AI4OPT Faculty Training Program, which focuses on strategies to increase minority participation in AI research programs from HBCUs to other minority-serving institutions," said Pierre, who also leads the Educational and Diversity Initiatives at AI4OPT. "Our goal is to ensure diverse representation in the AI field."
The collaboration will use existing educational resources and infrastructure to build centers of excellence in AI and a community of empowered Black AI researchers.
"We anticipate challenges in developing coursework, including finding qualified industry professionals to teach and preparing academic professors unfamiliar with AI," Pierre said. "Our aim is to establish Ph.D. programs at CAU and position the university as a hub for AI training, addressing these issues head-on."
AIHUB@CAU will integrate industry partnerships to accelerate curriculum development and real-world applications. It expands AI education beyond machine learning to encompass decision-making and applications in fields like business analytics, cyber-physical security, and operations research.
Partially funded through NSF's Louis Stokes Alliances for Minority Participation program, this award underscores NSF's commitment to diversity in STEM fields through impactful educational and research initiatives.
"Establishing programs at institutions like Clark Atlanta University and AI4OPT at Georgia Tech provides students with essential resources and tools to succeed in this ever-evolving field," Pierre noted.
Goals and Structure of the AI Education Program
Main Goals of Creating AI Courses at the Undergraduate and Graduate Levels:
- Close the gap of AI graduates from HBCUs at undergraduate and graduate levels.
- Prepare HBCU students for the AI workforce.
- Align with the vision of AI4OPT at Georgia Tech to "democratize access to AI education."
Impact on Students' Career Prospects and the AI Research Community:
- Undergraduate courses and programs will prepare students for entry-level positions in the field.
- Graduate courses and programs will prepare students for research and participation in the AI research community.
Role and Contribution:
- AI4OPT at Georgia Tech will assist CAU with the development of both undergraduate and graduate courses and programs.
- Offer research opportunities to CAU students at the undergraduate and graduate levels.
- AI4OPT at Georgia Tech will be a partner in the established AI Research Hub.
Support for Development of MS and Ph.D. Courses:
- Use current courses at Georgia Tech as a template.
- Use the courses offered through the Faculty Training Program (FTP) of AI4OPT.
Foundational AI Courses:
- Courses already taught by CAU faculty in the AI4OPT FTP.
- Courses available at Georgia Tech.
- New courses to be developed by AIHUB@CAU based on Intel material, focusing on computer vision and natural language processing.
- Courses in applied optimization developed by AI4OPT.
- New use-inspired AI courses teaching applications of AI in various domains, such as supply chains, security, chemistry, and manufacturing.
Research Opportunities:
- The Undergraduate Research Program (URP) will provide students with early exposure to AI research, including summer internships at Georgia Tech and other AI4OPT sites.
- The graduate programs will include an 18-month non-thesis master's degree with a summer internship and capstone project, and a two-year thesis master's degree supported by a six-month research project.
Structure of the New Master in AI Program:
- Courses in five categories to support the master’s program:
- Existing courses at CAU taught in the AI4OPT FTP.
- Courses available at Georgia Tech.
- New courses based on Intel material.
- Applied optimization courses developed by AI4OPT.
- New courses developed by AIHUB@CAU focusing on AI applications in various domains.
Collaborations and Internships:
- Joint supervision of research projects by CAU and AI4OPT faculty.
- Summer internships starting in 2026.
- Capstone projects facilitated by Georgia Tech and industrial partners.
About AI4OPT
The Artificial Intelligence (AI) Research Institute for Advances in Optimization, or AI4OPT, aims to deliver a paradigm shift in automated decision-making at massive scales by fusing AI and Mathematical Optimization (MO) to achieve breakthroughs that neither field can achieve independently. The Institute is driven by societal challenges in energy, logistics and supply chains, resilience and sustainability, and circuit design and control. To address the widening gap in job opportunities, the Institute delivers an innovative longitudinal education and workforce development program.
About Georgia Tech
The Georgia Institute of Technology, or Georgia Tech, is a top 10 public research university developing leaders who advance technology and improve the human condition. The Institute offers business, computing, design, engineering, liberal arts, and sciences degrees. Its nearly 40,000 students, representing 50 states and 149 countries, study at the main campus in Atlanta, at international campuses, and through distance and online learning. As a leading technological university, Georgia Tech is an engine of economic development for Georgia, the Southeast, and the nation, conducting more than $1 billion in research annually for government, industry, and society.
About Clark Atlanta University
Clark Atlanta University was formed with the consolidation of Atlanta University and Clark College, both of which hold unique places in the annals of African American history. Atlanta University, established in 1865 by the American Missionary Association, was the nation’s first institution to award graduate degrees to African Americans. CAU is also the largest of the 37-member UNCF institutions. CAU, established four years later in 1869, was the nation’s first four-year liberal arts college to serve a primarily African American student population. Today, with over 4,000 students, CAU is the largest of the four institutions (CAU, Morehouse College, Spelman College, and Morehouse School of Medicine) that comprise the Atlanta University Center Consortium.
About National Science Foundation
The U.S. National Science Foundation propels the nation forward by advancing fundamental research in all fields of science and engineering. NSF supports research and people by providing facilities, instruments and funding to support their ingenuity and sustain the U.S. as a global leader in research and innovation. With a fiscal year 2023 budget of $9.5 billion, NSF funds reach all 50 states through grants to nearly 2,000 colleges, universities and institutions. Each year, NSF receives more than 40,000 competitive proposals and makes about 11,000 new awards. Those awards include support for cooperative research with industry, Arctic and Antarctic research and operations, and U.S. participation in international scientific efforts.
Breon Martin
AI Research Communications Manager
Georgia Tech
Mentoring the Future of Nanotechnology
Jul 19, 2024 — Atlanta, GA
Marissa Moore and Blair Brettmann in the lab. Credit: Allison Carter
When Blair Brettmann was a sophomore at the University of Texas at Austin, her advisor told her about the National Science Foundation’s Research Experience for Undergraduates (REU) program. The summer program enables undergraduates to conduct research at top institutions across the country. Brettmann spent the summer of 2005 at Cornell working in a national nanotechnology program — a defining experience that led to her current research in molecular engineering for integrated product development.
“I didn't know for sure if I wanted to attend grad school until after the REU experience,” Brettmann said. “Through it, I went to high-level seminars for the first time, and working in a cleanroom was super cool.”
Her experience was so positive that the following summer, Brettmann completed a second REU at the Massachusetts Institute of Technology, where she eventually earned her Ph.D. Now an associate professor in Georgia Tech’s School of Chemical and Biomolecular Engineering and School of Materials Science and Engineering and an Institute for Matter and Systems faculty member, Brettmann is an REU mentor for the current iteration of the nanotechnology program — now taking place at Georgia Tech.
Brettmann’s mentee this summer, Marissa Moore, is having a similarly positive experience. A rising senior in chemical engineering at the University of Missouri-Columbia (Mizzou), Moore was already familiar with Georgia Tech because her father received his chemical engineering Ph.D. from the Institute; she hopes to do the same. Her passion for research began as she grew up with her sister, who had cerebral palsy and epilepsy.
“We spent a lot of time in hospitals trying out new devices and looking for different medications that would help her, so I knew I wanted to make a difference in this area,” she said.
But Moore wasn’t interested in being a doctor. Instead, she wanted to develop the materials that could be a solution for someone like her sister. Her undergraduate research focuses on materials and biomaterials for medical applications, and Georgia Tech is enabling her to deep-dive into pure materials science.
“What I'm working on at both universities is biodegradable polymers, but at Mizzou I’m developing that polymer from the ground up, and at Tech I’m using the properties of the polymer and finding how to make them,” she explained.
Having the opportunity to work in nanotechnology through the Institute for Materials and use Georgia Tech’s famous cleanroom made this REU stand out for Moore.
“I had never been in the cleanroom before, so that was one of the most eye-opening experiences,” she said. “It was cool to gown up and learn all of the safety precautions.”
For Brettmann, hands-on research experiences like this make the REU program unique — and crucial — for potential graduate students.
“Having your experiments fail, or even having things not turn out as you expect them to is an important part of the graduate research experience,” she said. “One of the best things about REU is it can be a first experience for people and help them decide what to do in grad school later on.”
Tess Malone, Senior Research Writer/Editor
tess.malone@gatech.edu
Georgia Tech Wins Second $25 Million Award to Support Nuclear Nonproliferation Research and Education
Jul 16, 2024 — Atlanta, GA

Photo by Joya Chapman
Georgia Tech will lead a consortium of 12 universities and 12 national labs as part of a $25 million U.S. Department of Energy National Nuclear Security Administration (NNSA) award. This is the second time Georgia Tech has won this award and led research and development efforts to aid NNSA’s nonproliferation, nuclear science, and security endeavors.
The Consortium for Enabling Technologies and Innovation (ETI) 2.0 will leverage the strong foundation of interdisciplinary, collaboration-driven technological innovation developed in the ETI Consortium funded in 2019. The technical mission of the ETI 2.0 team is to advance technologies across three core disciplines: data science and digital technologies in nuclear security and nonproliferation, precision environmental analysis for enhanced nuclear nonproliferation vigilance and emergency response, and emerging technologies. They will be advanced by research projects in novel radiation detectors, algorithms, testbeds, and digital twins.
“What we're trying to do is bring those emergent technologies that are not implemented right now to fruition,” said Anna Erickson, Woodruff Professor and associate chair for research in the George W. Woodruff School of Mechanical Engineering, who leads both grants. “We want to understand what's ahead in the future for both the technology and the threats, which will help us determine how we can address it today.”
While half the original collaborators remain, Erickson sought new institutional partners for their research expertise, including Abilene Christian University, University of Alaska Fairbanks, Stony Brook University, Rensselaer Polytechnic Institute, and Virginia Commonwealth University. Other university collaborators include the Colorado School of Mines, the Massachusetts Institute of Technology, Ohio State University, Texas A&M University, the University of Texas at Austin, and the University of Wisconsin–Madison.
National lab partners are the Argonne National Laboratory, Brookhaven National Laboratory, Idaho National Laboratory, Lawrence Berkeley National Laboratory, Lawrence Livermore National Laboratory, Los Alamos National Laboratory, Nevada National Security Site, Oak Ridge National Laboratory, Pacific Northwest National Laboratory, Princeton Plasma Physics Laboratory, Sandia National Laboratories, and Savannah River National Laboratory.
The partners, along with the other NNSA Consortia, gathered at Texas A&M in June to present the new results of the research — NNSA DNN R&D University Program Review — and the kickoff will be hosted in Atlanta in February 2025. More than 300 collaborators, including 150 students, met for four days to share their research and develop new partnerships.
Engaging students in research in the nuclear nonproliferation field is a key part of the award. The plan is to train over 50 graduate students, provide internships for graduate and undergraduate students, and offer faculty-student lab visit fellowships. This pipeline aims to develop well-rounded professionals equipped with the expertise to tackle future nonproliferation challenges.
“Because nuclear proliferation is a multifaceted problem, we try to bring together people from outside nuclear engineering to have a conversation about the problems and solutions,” Erickson said.
“One of the biggest accomplishments of ETI 1.0 is this incredible relationship that our university PIs have been able to forge with national labs,” she said. “Over five years, we've supported over 70 student internships at national labs, and we have already transitioned a number of Ph.D. students to careers at national labs.”
As the consortium efforts continue, the team looks forward to the next phase of engagement with government, university, and national lab partners.
“With a united team and a focus on cutting-edge technologies, the ETI 2.0 consortium is poised to break new ground in nuclear nonproliferation,” Erickson said. “Collaboration is the fuel, and innovation is the engine.”
Tess Malone, Senior Research Writer/Editor
tess.malone@gatech.edu
Using Deep Learning Techniques to Improve Liver Disease Diagnosis and Treatment
Jul 15, 2024 —

Professor Jun Ueda in the George W. Woodruff School of Mechanical Engineering and robotics Ph.D. student Heriberto Nieves.
Hepatic, or liver, disease affects more than 100 million people in the U.S. About 4.5 million adults (1.8%) have been diagnosed with liver disease, but it is estimated that between 80 and 100 million adults in the U.S. have undiagnosed fatty liver disease in varying stages. Over time, undiagnosed and untreated hepatic diseases can lead to cirrhosis, a severe scarring of the liver that cannot be reversed.
Most hepatic diseases are chronic conditions that will be present over the life of the patient, but early detection improves overall health and the ability to manage specific conditions over time. Additionally, assessing patients over time allows for effective treatments to be adjusted as necessary. The standard protocol for diagnosis, as well as follow-up tissue assessment, is a biopsy after the return of an abnormal blood test, but biopsies are time-consuming and pose risks for the patient. Several non-invasive imaging techniques have been developed to assess the stiffness of liver tissue, an indication of scarring, including magnetic resonance elastography (MRE).
MRE combines elements of ultrasound and MRI imaging to create a visual map showing gradients of stiffness throughout the liver and is increasingly used to diagnose hepatic issues. MRE exams, however, can fail for many reasons, including patient motion, patient physiology, imaging issues, and mechanical issues such as improper wave generation or propagation in the liver. Determining the success of MRE exams depends on visual inspection of technologists and radiologists. With increasing work demands and workforce shortages, providing an accurate, automated way to classify image quality will create a streamlined approach and reduce the need for repeat scans.
Professor Jun Ueda in the George W. Woodruff School of Mechanical Engineering and robotics Ph.D. student Heriberto Nieves, working with a team from the Icahn School of Medicine at Mount Sinai, have successfully applied deep learning techniques for accurate, automated quality control image assessment. The research, “Deep Learning-Enabled Automated Quality Control for Liver MR Elastography: Initial Results,” was published in the Journal of Magnetic Resonance Imaging.
Using five deep learning training models, an accuracy of 92% was achieved by the best-performing ensemble on retrospective MRE images of patients with varied liver stiffnesses. The team also achieved a return of the analyzed data within seconds. The rapidity of image quality return allows the technician to focus on adjusting hardware or patient orientation for re-scan in a single session, rather than requiring patients to return for costly and timely re-scans due to low-quality initial images.
This new research is a step toward streamlining the review pipeline for MRE using deep learning techniques, which have remained unexplored compared to other medical imaging modalities. The research also provides a helpful baseline for future avenues of inquiry, such as assessing the health of the spleen or kidneys. It may also be applied to automation for image quality control for monitoring non-hepatic conditions, such as breast cancer or muscular dystrophy, in which tissue stiffness is an indicator of initial health and disease progression. Ueda, Nieves, and their team hope to test these models on Siemens Healthineers magnetic resonance scanners within the next year.
Publication
Nieves-Vazquez, H.A., Ozkaya, E., Meinhold, W., Geahchan, A., Bane, O., Ueda, J. and Taouli, B. (2024), Deep Learning-Enabled Automated Quality Control for Liver MR Elastography: Initial Results. J Magn Reson Imaging. https://doi.org/10.1002/jmri.29490
Prior Work
Robotically Precise Diagnostics and Therapeutics for Degenerative Disc Disorder
Related Material
Editorial for “Deep Learning-Enabled Automated Quality Control for Liver MR Elastography: Initial Results”
Christa M. Ernst |
Research Communications Program Manager |
Topic Expertise: Robotics, Data Sciences, Semiconductor Design & Fab |
Will the Seine River’s E. coli Woes Sink Olympic Dreams in Paris?
Jul 15, 2024 —

Time is winding down on Olympic organizers’ plans to stage open-water swimming events in Paris’ iconic Seine River later this month. The city spent $1.5 billion on new infrastructure to clean up the Seine, yet water samples continue to show high levels of potentially toxic E. coli.
The river has been closed to swimmers for the past 100 years because of pollution, but Olympic organizers hope to stage the triathlon and marathon swimming events in the water flowing in the shadow of the Eiffel Tower.
Katherine Graham has followed the saga in Paris. She’s an assistant professor in the Georgia Tech School of Civil and Environmental Engineering who studies the fate and transport of pathogens and their indicators in water, including E. coli. She said several factors are at play in the Seine.
“Paris, like most large cities, has a lot of concrete and not much dirt and grass for water to soak into."
Read the entire story on the College of Engineering website.
Jason Maderer
College of Engineering
maderer@gatech.edu
A New Neural Network Makes Decisions Like a Human Would
Jul 15, 2024 —

Getty
Humans make nearly 35,000 decisions every day, from whether it’s safe to cross the road to what to have for lunch. Every decision involves weighing the options, remembering similar past scenarios, and feeling reasonably confident about the right choice. What may seem like a snap decision actually comes from gathering evidence from the surrounding environment. And often the same person makes different decisions in the same scenarios at different times.
Neural networks do the opposite, making the same decisions each time. Now, Georgia Tech researchers in Associate Professor Dobromir Rahnev’s lab are training them to make decisions more like humans. This science of human decision-making is only just being applied to machine learning, but developing a neural network even closer to the actual human brain may make it more reliable, according to the researchers.
In a paper in Nature Human Behaviour, “The Neural Network RTNet Exhibits the Signatures of Human Perceptual Decision-Making,” a team from the School of Psychology reveals a new neural network trained to make decisions similar to humans.
Decoding Decision
“Neural networks make a decision without telling you whether or not they are confident about their decision,” said Farshad Rafiei, who earned his Ph.D. in psychology at Georgia Tech. “This is one of the essential differences from how people make decisions.”
Large language models (LLM), for example, are prone to hallucinations. When an LLM is asked a question it doesn’t know the answer to, it will make up something without acknowledging the artifice. By contrast, most humans in the same situation will admit they don’t know the answer. Building a more human-like neural network can prevent this duplicity and lead to more accurate answers.
Making the Model
The team trained their neural network on handwritten digits from a famous computer science dataset called MNIST and asked it to decipher each number. To determine the model’s accuracy, they ran it with the original dataset and then added noise to the digits to make it harder for humans to discern. To compare the model performance against humans, they trained their model (as well as three other models: CNet, BLNet, and MSDNet) on the original MNIST dataset without noise, but tested them on the noisy version used in the experiments and compared results from the two datasets.
The researchers’ model relied on two key components: a Bayesian neural network (BNN), which uses probability to make decisions, and an evidence accumulation process that keeps track of the evidence for each choice. The BNN produces responses that are slightly different each time. As it gathers more evidence, the accumulation process can sometimes favor one choice and sometimes another. Once there is enough evidence to decide, the RTNet stops the accumulation process and makes a decision.
The researchers also timed the model’s decision-making speed to see whether it follows a psychological phenomenon called the “speed-accuracy trade-off” that dictates that humans are less accurate when they must make decisions quickly.
Once they had the model’s results, they compared them to humans’ results. Sixty Georgia Tech students viewed the same dataset and shared their confidence in their decisions, and the researchers found the accuracy rate, response time, and confidence patterns were similar between the humans and the neural network.
“Generally speaking, we don't have enough human data in existing computer science literature, so we don't know how people will behave when they are exposed to these images. This limitation hinders the development of models that accurately replicate human decision-making,” Rafiei said. “This work provides one of the biggest datasets of humans responding to MNIST.”
Not only did the team’s model outperform all rival deterministic models, but it also was more accurate in higher-speed scenarios due to another fundamental element of human psychology: RTNet behaves like humans. As an example, people feel more confident when they make correct decisions. Without even having to train the model specifically to favor confidence, the model automatically applied it, Rafiei noted.
“If we try to make our models closer to the human brain, it will show in the behavior itself without fine-tuning,” he said.
The research team hopes to train the neural network on more varied datasets to test its potential. They also expect to apply this BNN model to other neural networks to enable them to rationalize more like humans. Eventually, algorithms won’t just be able to emulate our decision-making abilities, but could even help offload some of the cognitive burden of those 35,000 decisions we make daily.
Tess Malone, Senior Research Writer/Editor
tess.malone@gatech.edu
HBCU CHIPS Network Defines Organization's Strategic Direction at Atlanta Meeting
Jul 14, 2024 —
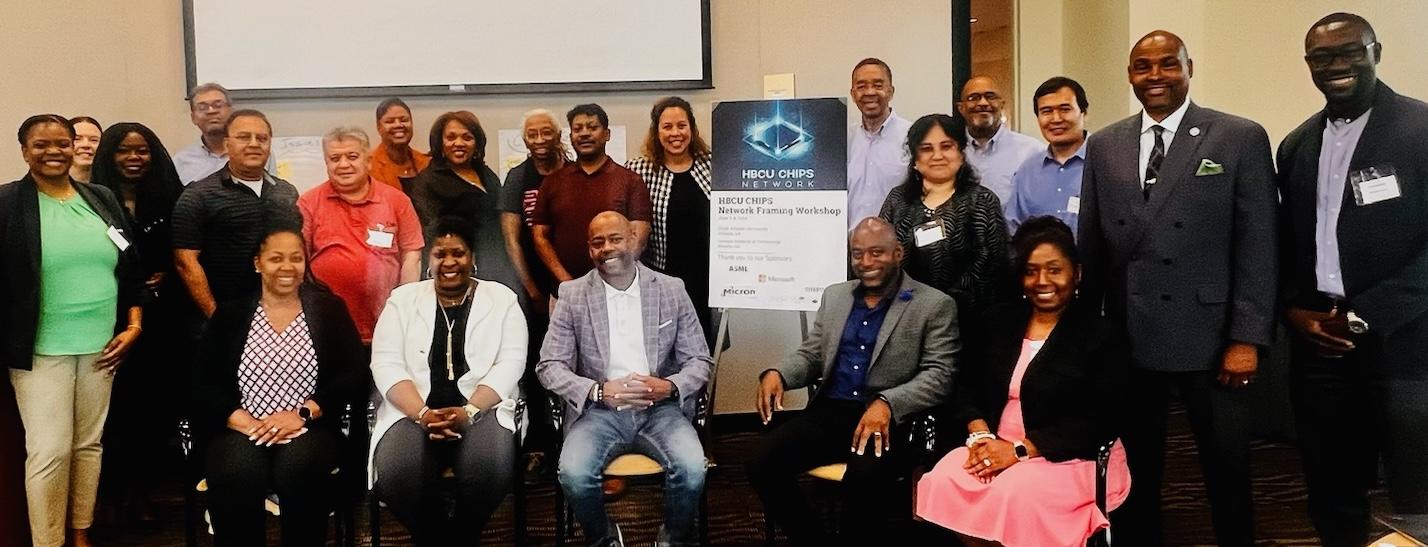
The consortium of historically Black educational institutions and other stakeholders convened to establish the organization’s strategic direction and governance model. The goal is to foster a diverse workforce and drive innovation in the U.S. semiconductor industry.
In the heart of Atlanta, members of the HBCU CHIPS Network gathered for a pivotal meeting on June 3-4, 2024. Taking place at Georgia Institute of Technology’s campus, 30-plus representatives from historically Black colleges and universities (HBCUs), historically Black community colleges (HBCCs), nonprofit organizations, and the Institute convened to chart a new course for the microelectronics industry.
At the event, co-hosted by Clark Atlanta University (CAU) and Georgia Tech, attendees worked to establish a strategic direction for the HBCU CHIPS Network, as well as a formal operational and governance model — with the principal goals of enhancing research collaboration, positioning for CHIPS Act funding, and empowering a diverse, inclusive workforce that can meet the needs of the growing U.S. semiconductor sector.
Dietra Trent, executive director for the White House Initiative on HBCUs, attended the first day’s sessions. Frances Williams, vice president for Research and Sponsored Programs at CAU and one of the organizers and co-leaders of the HBCU CHIPS Network, welcomed the attendees and outlined the meeting agenda.
Over two days of discussion, facilitated by Michael Wilkinson, the founder of Leadership Strategies, members reframed the Network’s vision as follows: “The HBCU CHIPS Network is envisioned as a research and education consortium that serves as the nexus of collaboration and cooperation between HBCUs, government agencies, academia, and industry … Through a multidisciplinary approach, the Network will facilitate fulfilling talent pipelines to grow the workforce of the future, research innovations, resolving longstanding disparities in facilities, building out domestic capacity, and providing shared accessibility across the Network stakeholders.”
The group also established the Network’s governance and operational model, including its leadership and organizational structure.
According to George White, senior director for Strategic Partnerships at Georgia Tech and an HBCU graduate, “The outcome from this workshop has the potential to transform HBCU research collaboration and innovation well beyond the CHIPS Act. Additionally, the Network will provide outreach to community colleges, veterans, and k-12 students, empowering a diverse and inclusive workforce that leverages research innovations, including experiential learning opportunities across all stakeholder groups.”
Nationally, the network comprises five regions: the Southeast, the mid-Atlantic and South Atlantic, the Midwest, and the Southwest. Each region will have representation commensurate with their competencies and capacities in microelectronics. Selected board members within each region will constitute the leadership structure. The Network will have affiliate members that include nonprofit organizations and academic institutions like Georgia Tech.
Further, the Network identified several committees and working groups, including technical advisory, education and workforce, innovation and entrepreneurship, contracting, facilities access, communication and tech transfer, and assessment and evaluation. These committees will meet regularly and communicate status and outcomes to the larger Network.
The Network plans to host an annual conference highlighting research from participating HBCUs/HBCCs and industry partners, and the event will include a student-focused career fair.
The HBCU CHIPS Network thanks ASML, Micron, Microsoft, and Synopsys for their sponsorship.
Taiesha Smith, Sr. Program Manager, HBCU-MSI Research Partnerships
taiesha.smith@gatech.edu