Yonggang Ke
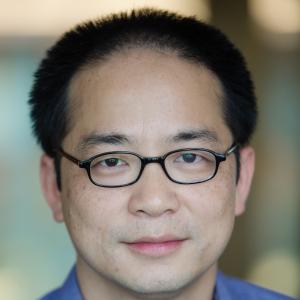
Yonggang Ke's research is highly interdisciplinary combining chemistry, biology, physics, material science, and engineering. The overall mission of his research is to use interdisciplinary research tools to program nucleic-acid-based "beautiful structures and smart devices" at nanoscale, and use them for scientific exploration and technological applications.